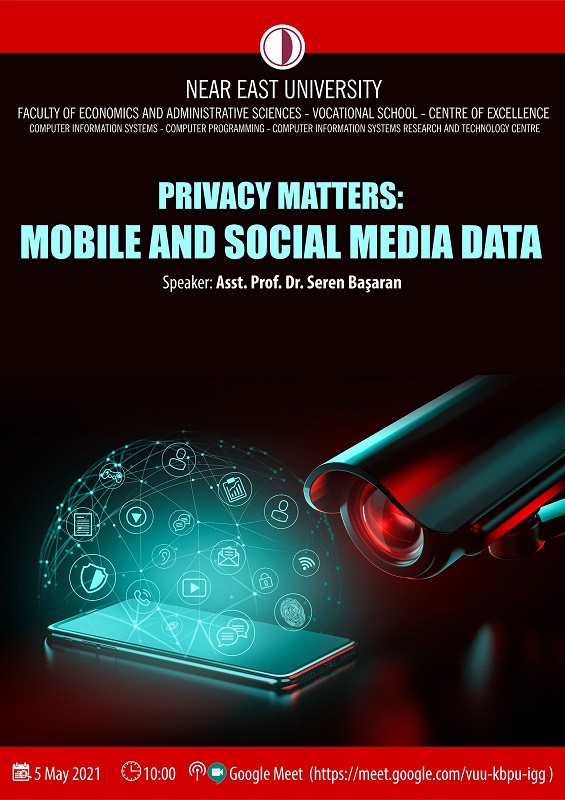
This talk presents an introduction to machine learning and data analytics, their possibilities and their limits, as well as examples of the application of the different developed approaches. The talk begins with an introduction to data analysis which presents the representation spaces and the different types of data. Next, the focus is on big data and the issues raised by these information resources whose characteristics in terms of volume, velocity, and variety, require the use of specific analytical methods and technologies to generate value. In this context, the applications will be approached by highlighting the encountered constraints in terms of storage, analysis, and computing capacities. After a brief history, the different machine learning approaches will be presented. Deep learning architectures such as deep neural networks, convolutional deep neural networks, and deep belief networks, will be presented. Evolution perspectives and a conclusion close this talk.